Online Survey Software
Discover what your customers and employees are really thinking
Survey software gets answers to your most important customer, employee, marketing and product questions. It can handle everything from simple customer feedback questionnaires to detailed research projects for the world’s biggest brands.
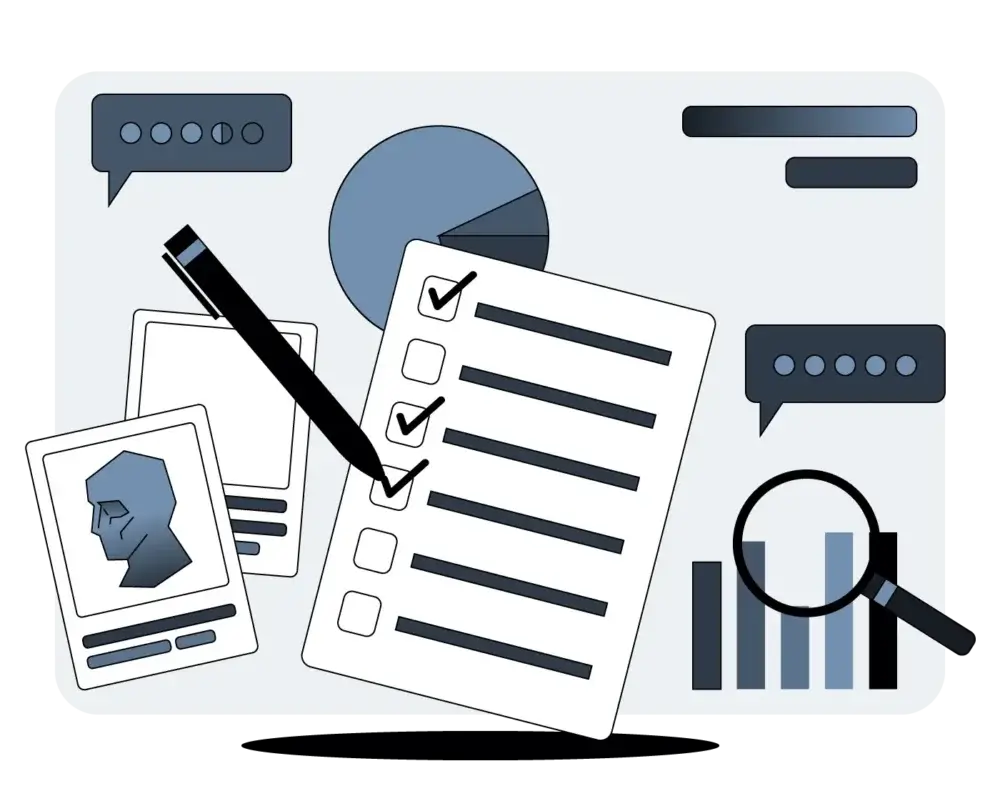
Today's reality—sound familiar?
2.6x more success could have been realized in marketing campaigns with better research & insights.
23% of organizations don’t have a clear market research strategy in place.
13% of marketing spend is wasted for reasons that could have been addressed through better market research.
With online survey software you can:
- Eliminate manual data collection
- Get real-time, actionable insights
- Reach more people, faster and easier
- Get better, more honest responses
- Create professional surveys without any experience
Ready to take your market research to the next level?
Answers and insights from your audience, wherever
they are
Wherever you need to gather data, survey software can help. From a simple survey link you can paste anywhere, to advanced integrations with your CRM, to email, social, website, QR code, SMS and offline surveys, we’ll help you reach your target respondents, no matter where they are.
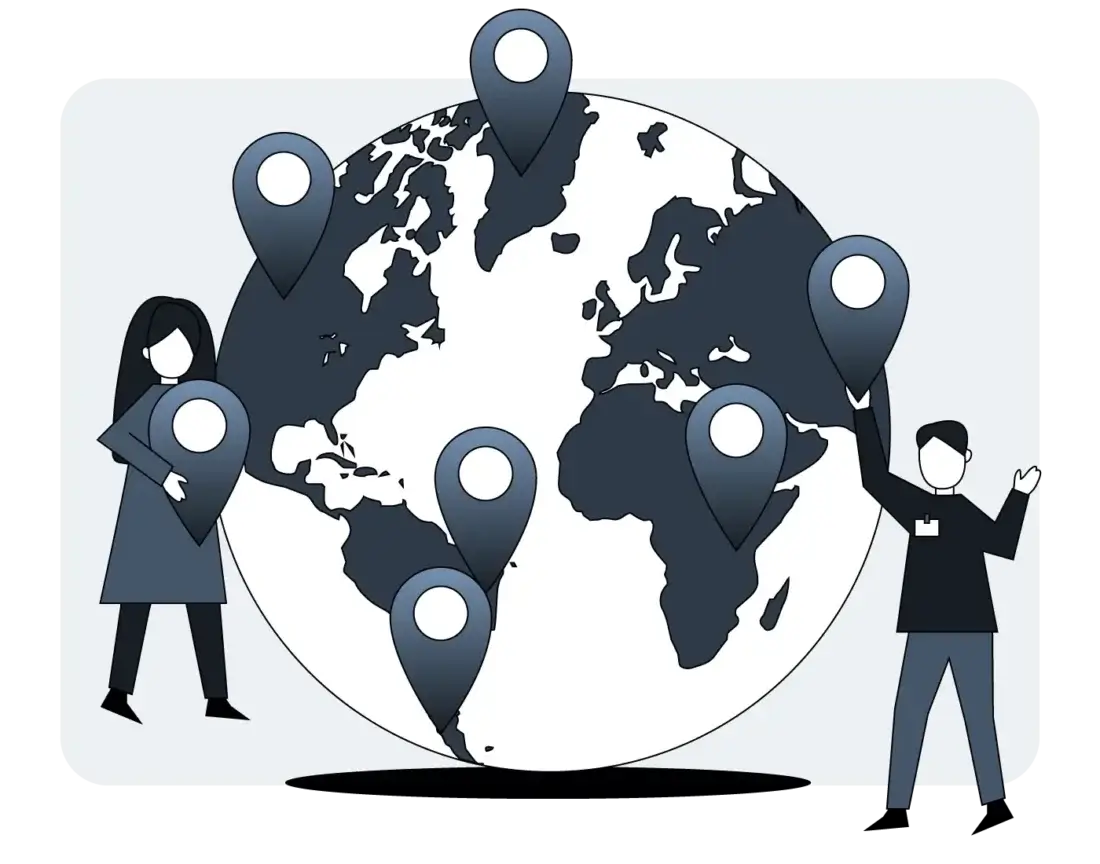
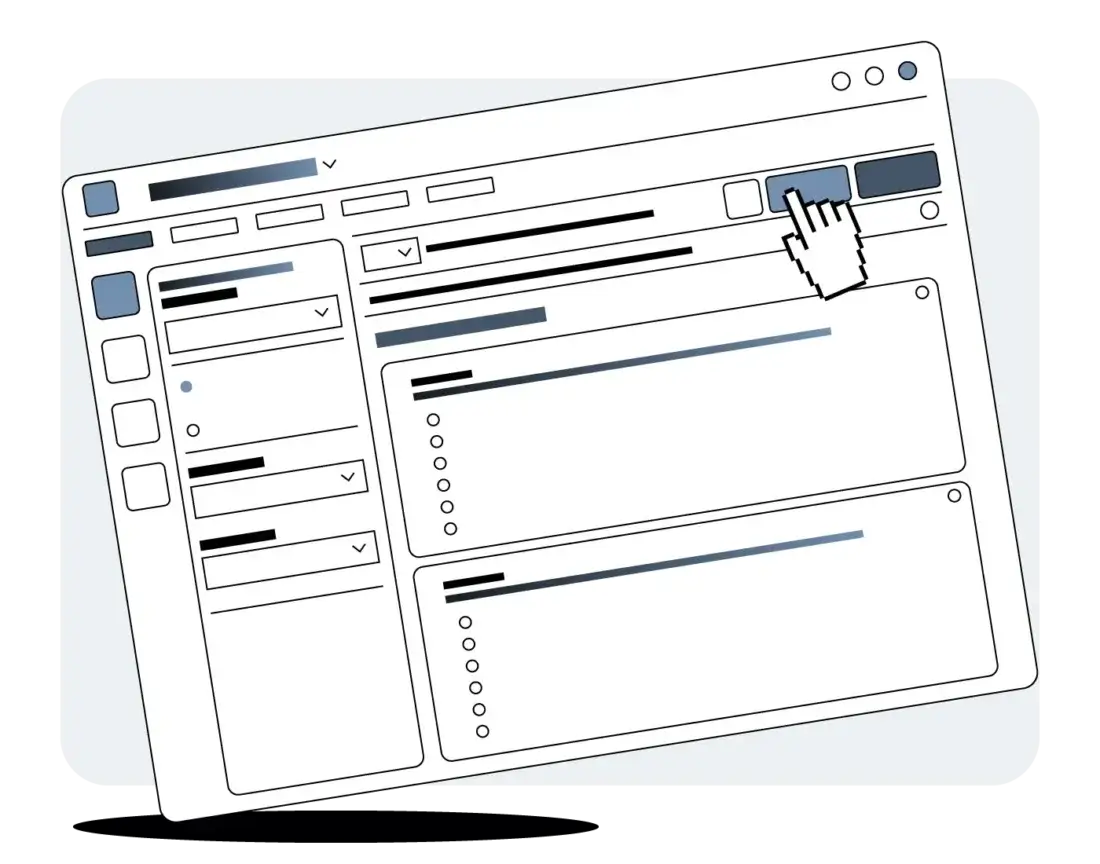
Drag-and-drop simplicity for even the most advanced surveys
Choose from 23 question types (including video/audio responses) and use advanced logic, branching, quotas, API integrations into Zendesk and email triggers to build and launch your project. It’s all done in an intuitive drag-and-drop software interface that makes even the most sophisticated surveys easy to create, launch and analyze.
Next-level survey reports and dashboards
Make better decisions with advanced reports and dashboards you can share in seconds. Choose from over 30 different graph types, share reports online, or export survey data to popular formats like CSV, TSV, Excel, SPSS and more.
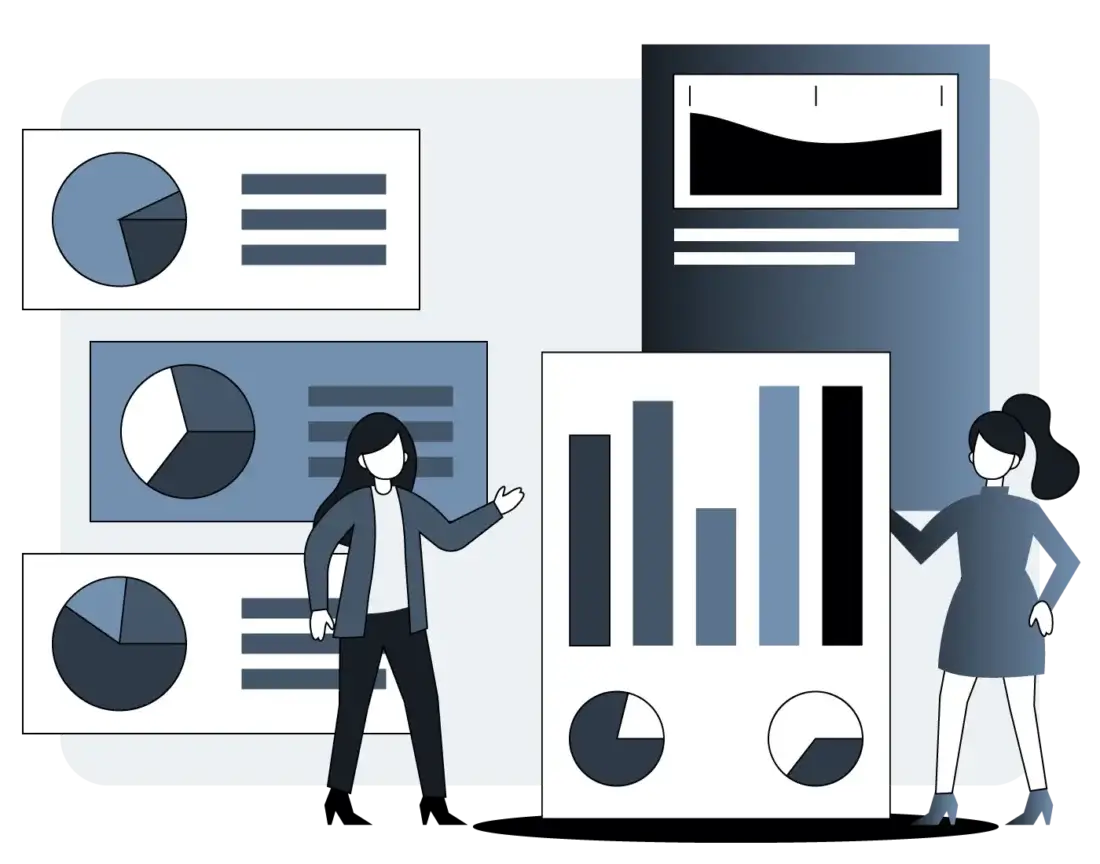
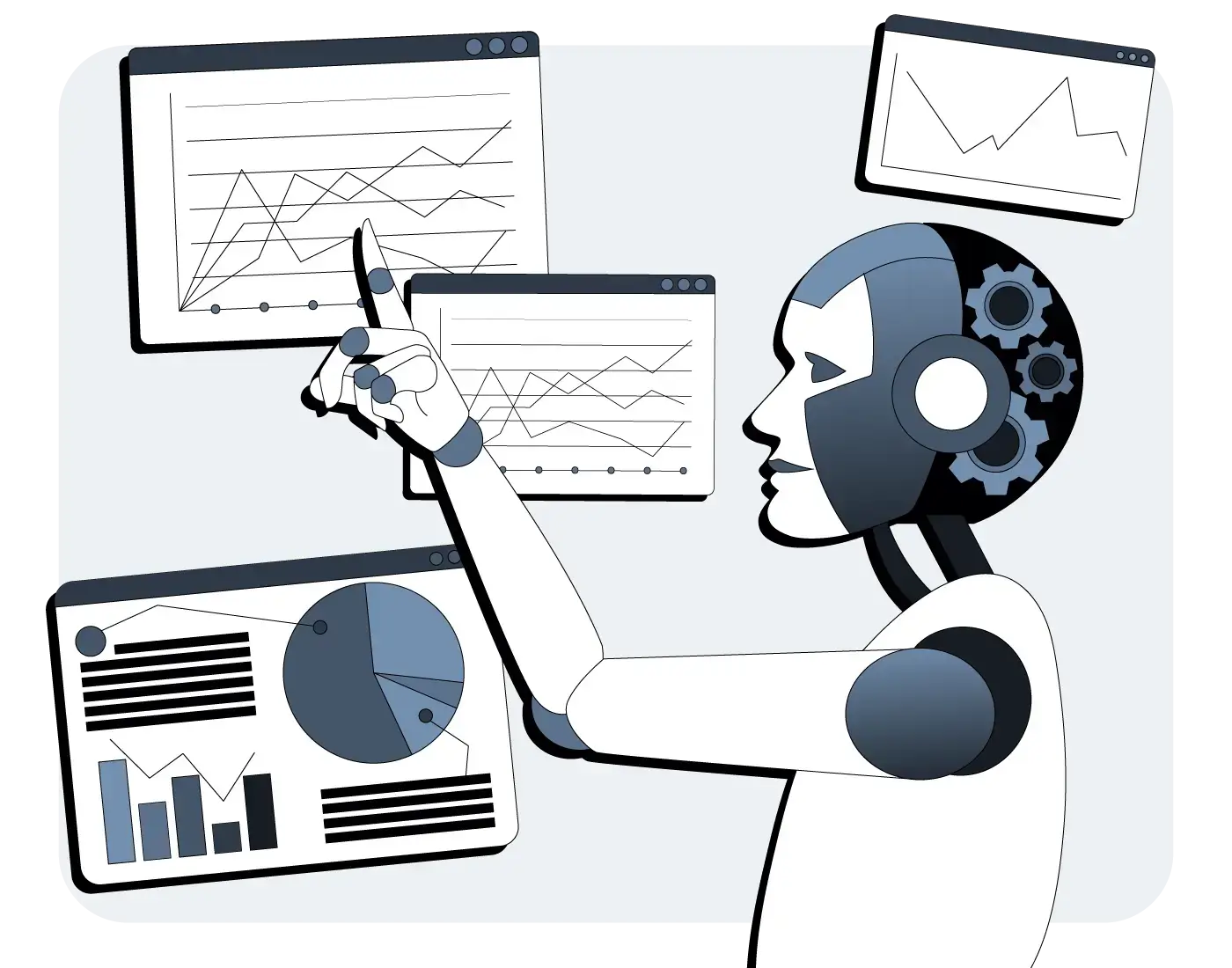
Built-in intelligence with every type of survey
Leverage advanced analysis, including video feedback summarization powered by generative AI, crosstabs, and statistical analysis tools. Automatically review survey design to ensure methodology best practices, response quality, and compliance with internal policies and PII.
Free survey templates
Net Promoter Score (NPS) Survey
Gauge your customers’
loyalty in seconds
Customer Satisfaction Survey
Unlock powerful insights on
your customers’ experience
Vote and Rank Survey
Prioritize decisions based
on crowd wisdom
Employee Engagement Survey
Keep pace with your workforce and
drive the outcomes that matter
Employee Satisfaction Survey
Measure employee satisfaction
and prioritize your resources
Product Research Survey Template
Test new products and features
with target customers
Employee Suggestion Box
Empower staff voices anonymously
for better business outcomes
Student Satisfaction Survey
Improve your educational offerings
and students’ experience
Quick Poll Template
Get instant results for
quick decisions
You’re in good company
Qualtrics has helped us bring some exciting new products to life, and ensured that we’re communicating the benefits in a way that resonates
Qualtrics enabled us to break silos that previously existed, helping us share customer insights across the group and reach our goals quicker
Ready to take your market research to the next level?
Survey software FAQs
A survey is a method of gathering information using relevant questions from a sample of people with the aim of understanding populations as a whole. Surveys provide a critical source of data and insights for everyone engaged in the information economy, from businesses to media, to government and academics.
Survey software is a tool used to design, send and analyze surveys online. It’s the primary method of collecting feedback at scale whether that’s a simple questionnaire or a detailed study such as customer or employee feedback as part of a more structured experience management program. Cloud-based survey technology has revolutionized the ability to get data, quickly, from a large number of respondents by automating the process of sending out surveys across a variety of channels from websites and mobile to apps, email and even chatbots.
Surveys provide quick, quantitative data on a wide audience’s opinions, preferences, and experiences. They are cost-effective, easy to administer, and can reach a large population. They also allow for anonymity, increasing the chance of honest responses, and their standardized format makes it easy to aggregate and analyze data for clear insights into trends and patterns.
To create a survey, define the objectives, choose target participants, design clear and concise questions, select a survey tool or platform, and ensure the layout is logical. Test the survey, distribute it, and collect responses. Remember to keep it as brief as possible while gathering the necessary information.
To write survey questions, be clear and specific to avoid confusion. Use simple, unbiased language, and opt for closed-ended questions for easier analysis. Ensure questions are relevant to your objectives, and avoid leading or loaded questions that could influence answers. Pretest your questions to catch any issues and revise as needed for clarity and objectivity.
Now used by more than 20,000+ brands, and supporting more than 1.3 billion surveys a year, Qualtrics empowers organizations to gather invaluable customer insights and take immediate, game-changing action – with zero coding required. The Qualtrics survey tool makes it easy to get answers to your most important marketing, branding, customer, and product questions, with easy-to-use tools that can handle everything from simple customer feedback questionnaires to detailed research projects.
Qualtrics Strategic Research pricing is based on interactions including number of survey responses and minutes of video feedback. Our special online pricing offer starts at $420 per month and can be purchased here. Alternatively, you can get started with a free account with basic functionality, or get 30 days access to advanced features with a free trial.
Yes, we offer a free account option with basic survey functionality.