Conjoint Analysis Software Tool
Optimize products and pricing with predictive insights
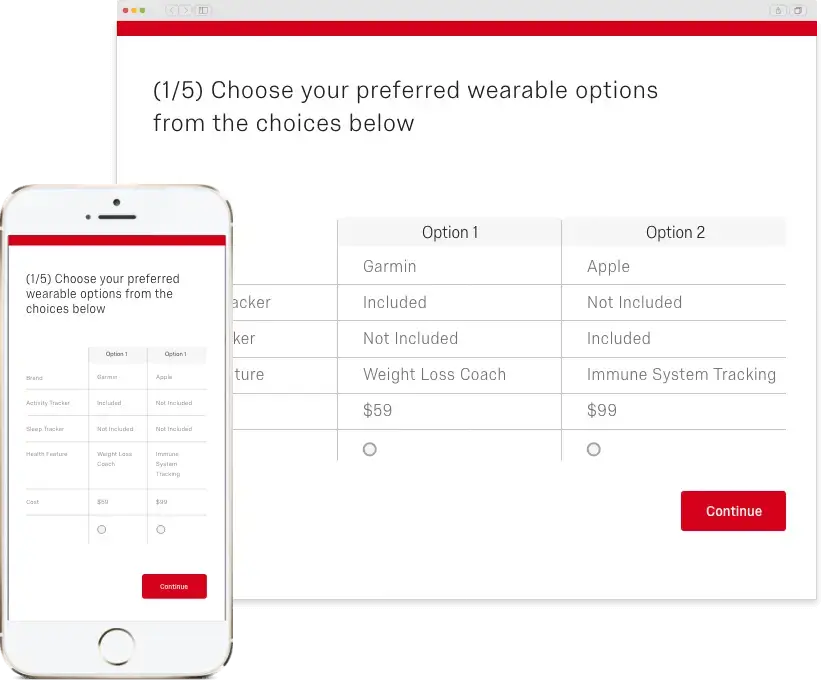
Over 16K brands and 99 of the top 100
business schools use Qualtrics
Take guesswork out of developing products and services customers will love. Whether you’re launching a new product or designing an employee benefits package, product optimization–powered by conjoint analysis automatically configures the product and price that will have the biggest impact on your business.
- Automatically discover the perfect product configuration and price before you launch
- Identify the priority areas for development to maximize your chances of success
- See the impact of changes to product features and pricing with the intuitive simulator and even benchmark against competitors
- Analyze your results in a few clicks for powerful, actionable insights
Make better decisions with Qualtrics and conjoint analysis
Get your products right in four simple steps
What used to take weeks of work and years of experience is now finished in minutes–no advanced research degree required. In four simple steps configure your study, review and field your survey and analyze the results in real time. Optimization automatically shows you the perfect product or service configuration at the right price.
Let your images do the talking
If a picture speaks a thousand words, Conjoint Dynamic Images speaks volumes. By using images instead of text-based attributes, you can quickly and easilytest combinations of visual product and package features to determine which ones your customers love. The result? Less survey fatigue, higher response rates and faster time to results.
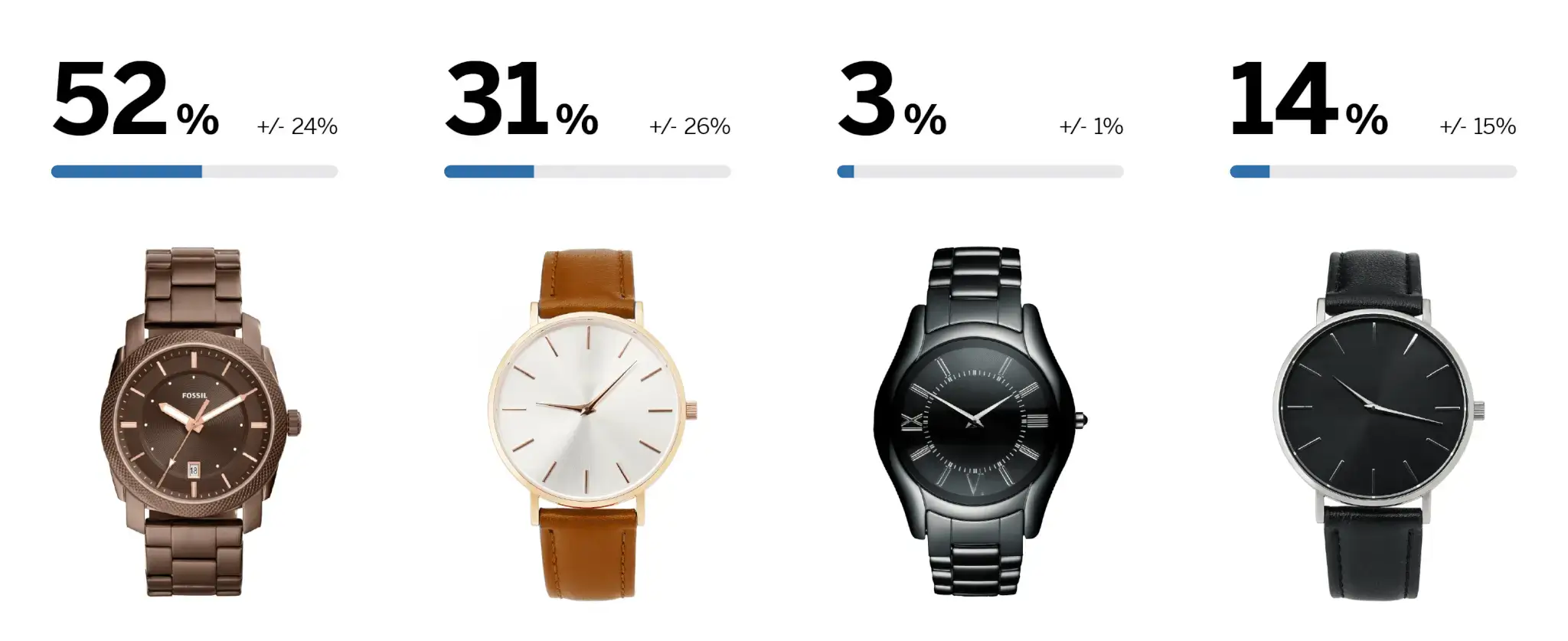
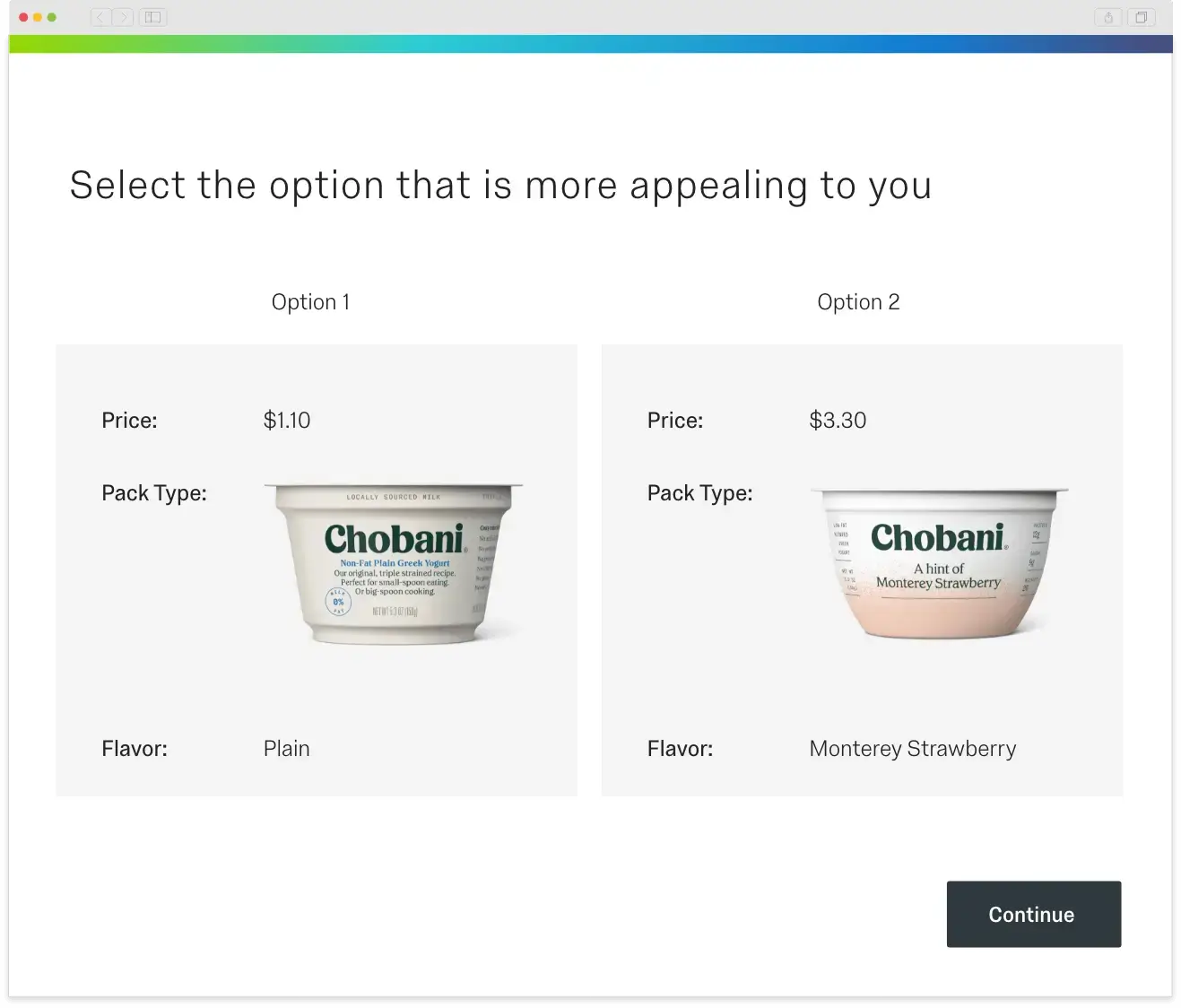
Put your product to the test
Automatically see the optimal product configuration and price sensitivity with the intuitive simulator. You can customize and compare different product configurations, benchmark against competitors’ products, and determine sensitivity to price and feature changes. You can even use the Qualtrics custom segmentation tool to zoom into specific segment attitudes towards different product configurations.
All the sophistication. None of the complication.
With Optimization, it’s all done for you–say goodbye to learning how to add attributes, feature sets, prohibited pairs, and manually calculating complicated hierarchical Bayesian estimation for utility scores. Our step-by-step guide makes it easy to add product features and launch a conjoint project in just minutes. And with all the complex stats automatically run in the background to produce the perfect product configuration and price for you, you’ll be building better products in no time.
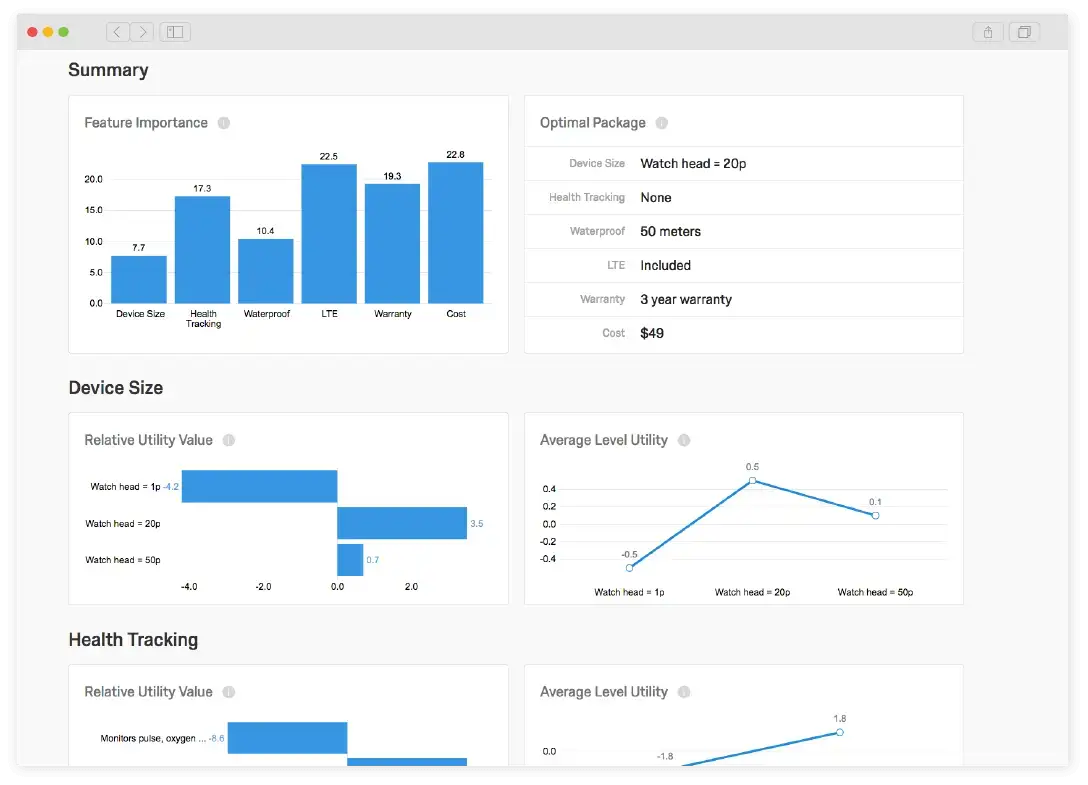
What is a conjoint analysis?
Conjoint analysis is a popular market research approach for measuring the value that consumers place on individual and packages of features of a product. The conjoint analysis approach combines real-life scenarios and statistical techniques with the modeling of actual market decisions. Conjoint analysis is commonly used in product testing and employee benefits packages. Conjoint surveys will show respondents a series of packages where feature variables are different to better understand which features drive purchase decisions.