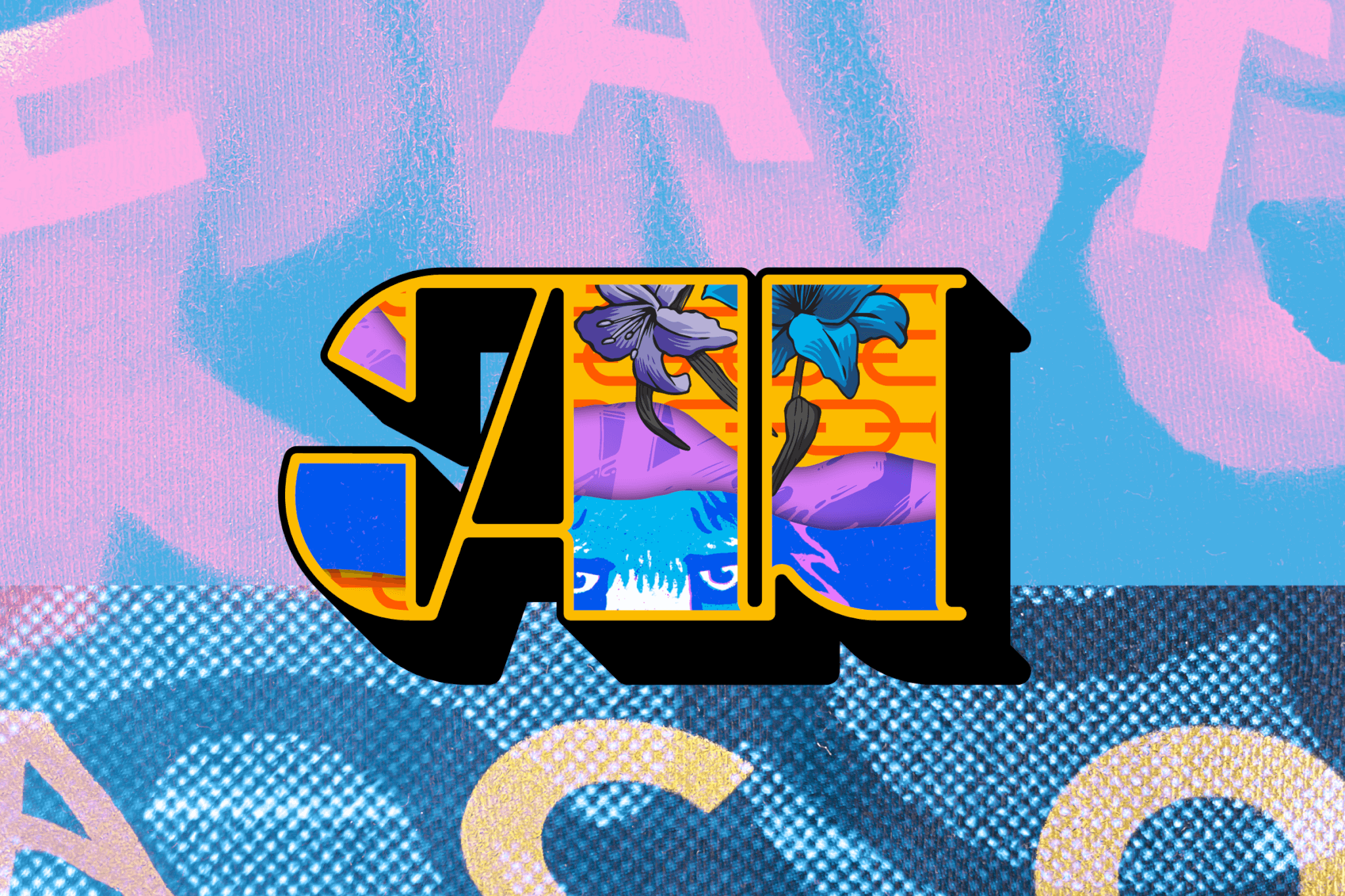
Experience Management
Artificial Intelligence is already transforming Experience Management — here’s where it goes next
It seems you can’t go a day without seeing another headline (or maybe 5!) about how Artificial Intelligence (AI) will transform how we live, work, and interact with the companies around us. But what is AI, how does it work in the XM Platform® and how will recent developments from OpenAI, Google, and others impact Experience Management (XM)?
There's no doubt about it: AI is transforming every moment of our lives. And we mean every moment.
Our inboxes are filled with requests from customers asking about our approach to new AI technologies, including those in the news, and what we’re doing to protect their data.
It’s not surprising, either — it seems like every week there's a new headline about ChatGPT and the profound changes AI will bring to how we work and live.
In the past 12 months, AI has moved from being the preserve of computer scientists and data science experts, and come into the mainstream thanks to OpenAI’s and Google’s releases of chatbot-style AI products.
It’s added fuel to the conversation by making these technologies easily accessible and significantly higher-quality than previous iterations, and captured people’s imaginations about how they could be applied to their work.
And while there is a ton of buzz right now about AI, their adoption must be accompanied by an increased focus on data privacy and security.
Sure, these new technologies will accelerate innovation, create new jobs and unlock new ways to get more value from XM, but their adoption must be accompanied by an increased focus on data privacy and security.
AI Jargon Buster
Before we go on, it’s worth a quick refresher on all the various terms you’ll have heard used when reading about AI.
- Generative Pre-trained Transformers (GPT) are a type of AI model that has been trained on large amounts of data and can generate human-like text, images, and even audio.
- Large Language Models (LLM) are similar to GPTs but are specifically designed for natural language tasks.
- Natural Language Processing (NLP) is a subset of AI that focuses on analyzing and understanding human language.
- Machine Learning (ML) is a technique used in AI where machines are trained to learn and improve on tasks without being explicitly programmed.
- Neural networks are a type of machine learning model that’s inspired by the structure of the human brain that processes and transmits information, enabling models to learn and make predictions based on input data.
- Deep learning is a subfield of machine learning that uses neural networks with multiple layers to solve complex problems such as recognizing patterns in data, making them well-suited for tasks such as image and speech recognition.
AI in Experience Management (XM) — the result of years of innovation
We’re already seeing the impact AI can have for our customers with the innovations we’ve rolled out on the XM Platform® over the past few years.
At the heart of those innovations lie machine learning (ML), and more specifically, deep learning — two areas we’ve been fine-tuning and innovating on for several years here at Qualtrics.
From combing through millions of online reviews, survey data, and various other listening mechanisms, you’ve probably already seen them in action in your own programs.
One of those machine learning mechanisms, Large Language Models (LLMs), has garnered a lot of attention recently as the foundational model behind ChatGPT, Bard, and many other publicly available AI.
We also leverage LLMs in our own AI, training them on the exact data they're expected to see ‘in the wild’ so they can deliver the kind of precision and accuracy our customers need.
Beyond LLMs, which today are often trained on broad and often widely disparate public data sources culled from the internet, our platform is informed by what we believe is the largest collection of human data experience on the planet - from calls, emails, feedback, social conversations, online reviews, chats, chatbot interactions, and more.
From these data sources we've developed over 150 models optimized for experience management across multiple industries, use cases, and customer types, regulatory domains, and more.
And then there’s our deep learning Natural Language Processing (NLP) model that understands sentiment, intent, empathy, and effort in any interaction, and in 23 languages (24 if you include emojis 😉).
These technologies have unlocked new features and capabilities for our customers, helping them make smarter decisions, stay connected to what matters, and take action that creates memorable experiences.
Our products offer a wide range of benefits for everyone in your organization. They help researchers and XM practitioners identify real signals from noisy data, provide contact center agents with the best guidance, and bring structured and unstructured data together to improve both employee and customer engagement and satisfaction.
And now, with the advances we’re seeing elsewhere in the industry, we’re excited about incorporating GPT into our home-grown AI to unlock even greater opportunities.
Keeping ethics, privacy, and function front of mind in our approach to AI
One challenge that’s garnered a lot of attention from both our customers, and also in the media, is the impact of publicly-available AI on data security.
That’s a challenge we take incredibly seriously as we balance the transformative potential provided by combining XM-trained AI with new, generative AI capabilities with our customers’ security and privacy.
As we incorporate AI tools into our tech stack, we make sure we have the appropriate contractual agreements in place to secure both our data and our customers’ data.
And we continually monitor industry and regulatory updates, including the use of personal and sensitive data in AI, to ensure that any new technologies we do roll out meet our customers’ evolving needs.
There’s no shortage of big, shiny objects that can capture our attention, and while it might be tempting to jump in with both feet to each new trend and technology, we must put our customers’ security first.
It’s an exciting future ahead for XM with AI
There’s no doubt about it, advancing the Qualtrics platform with new AI capabilities will help you take your XM programs to the next level.
Earlier this year at X4 in Salt Lake City, we demonstrated how ChatGPT could be used to describe a large dataset quickly and accurately from a research study.
For customers challenged with doing more with fewer resources and smaller teams, this new technology could be game-changing. By automating report compilation, they can free up bandwidth to focus on developing innovative ideas that will propel their business forward.
That’s just one use case where we see huge potential to combine the power and precision of the XM-trained AI we’re developing at Qualtrics with the innovations being rolled out by organizations like OpenAI.
And of course, we know it’s not about blindly adopting a single company or technology. Our commitment is to carefully evaluate the innovations, both in-house and from leading innovators in the field, and to identify the ones that provide the best value to our customers while prioritizing security, compliance, and data privacy.
See how AI is driving a new wave of XM innovations