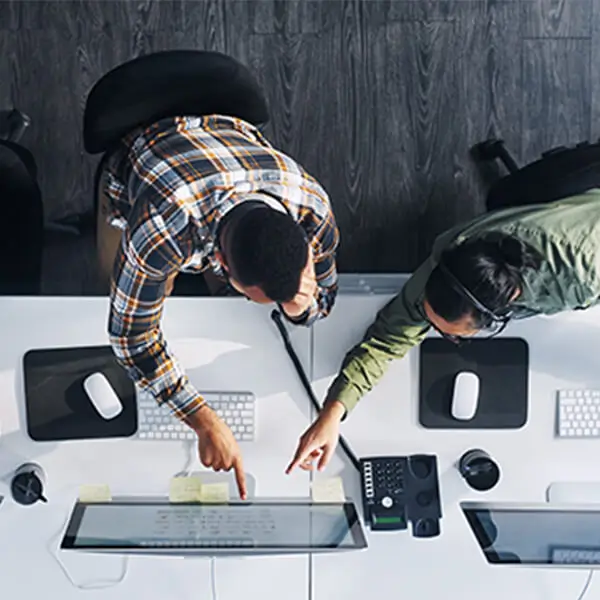
Customer Experience
How to incorporate three categories of AI into your XM efforts
While artificial intelligence (AI) promises to reshape Experience Management (XM) efforts, turning its potential into reality remains a challenge for organizations, many of whom remain stuck in “pilot purgatory.” This article breaks down three core categories of AI – Analytical, Generative, and Agentic – and provides practical guidance for embedding AI within your customer or employee experience program. This guidance should help you capture your share of the $860 billion AI opportunity while building advantages that competitors can't easily replicate.
The State of AI for Experience Management
Many traditional approaches to Experience Management – like periodic surveys, manual analyses, static reports – struggle to keep up with modern business demands. Organizations today must navigate an increasingly uncertain and complex landscape, defined by challenges like declining customer loyalty and employee intent to stay, accelerating technological change, and rising market volatility. Artificial intelligence has emerged as a catalyst, helping XM teams not only overcome these constraints but fundamentally reimagine how their organizations understand and serve customers and employees.
In fact, AI has the potential to drive an estimated $860 billion – and up to $1.3 trillion – in annual value by enabling organizations to develop richer insights, deliver more personalized experiences, and operate more efficiently at scale. Executives increasingly recognize this opportunity. Nearly three in four expect AI to transform their approach to customer and employee experience within just three years, and 69% believe it will significantly or completely change how their industry operates over the same timeframe.
Yet despite widespread recognition of AI's potential, few organizations are making the changes necessary to capitalize on this opportunity. While 89% of organizations have launched AI initiatives, most remain trapped in what we call "pilot purgatory," a state of fragmented experimentation that leaves them unable to scale their efforts or achieve meaningful results. Only 12% have established an organization-wide strategy with centralized ownership, but those that have are 2.3x more likely to report market share gains compared to their peers.
This gap between AI expectation and execution is creating a widening divide in the market. Because AI capabilities become smarter and more valuable with every interaction, organizations that move decisively now are building advantages that competitors will find increasingly difficult to overcome. Meanwhile, those that fail to move beyond isolated experiments risk watching their market position erode as competitors offer AI-enabled experiences that continuously improve and scale.
The Three Categories of AI
The widening gap between AI leaders and laggards creates mounting pressure for Experience Management teams to act. While most customer experience (CX) and employee experience (EX) leaders recognize AI's transformative potential, many struggle to navigate the rapidly evolving technology landscape and translate technical capabilities into practical applications.
Rather than getting lost in technological complexity or chasing the latest AI headlines, we recommend focusing on three fundamental categories of AI that can advance core Experience Management activities:
- Analytical AI. Capabilities that help organizations understand what's happening and why by finding patterns and deriving insights from data.
- Generative AI. Capabilities that help organizations communicate more effectively at scale by creating relevant content and contextual responses.
- Agentic AI. Capabilities that help organizations take coordinated action by orchestrating complex processes across systems to achieve specific experience goals.
As these capabilities mature and become embedded in operational workflows, organizations won't just incrementally improve their XM capabilities – they will fundamentally revolutionize how they understand and create value for customers, employees, and the business, building sustainable competitive advantages that competitors can't easily replicate.
Analytical AI
Analytical AI transforms vast amounts of data into actionable intelligence through technologies that combine advanced statistical analysis and machine learning models. These capabilities help organizations understand past events, predict future outcomes, and surface data-driven opportunities. By processing both structured and unstructured data, these systems automatically uncover patterns and insights that would be difficult, if not impossible, for humans to discover through conventional methods.
Applications for Analytical AI in XM include:
- Sentiment and Theme Analysis. Analytical AI applies natural language processing (NLP) and machine learning algorithms to unstructured feedback, automatically detecting patterns in sentiment, themes, and emotional signals across communication channels. A state government agency could analyze millions of citizen interactions across department websites, service centers, and social media to identify systemic pain points, surface demographic-specific communication gaps, and prioritize high-impact improvement opportunities, helping it drive measurable gains in service delivery effectiveness and citizen satisfaction.
- Predictive Attitude and Behavior Modeling. Analytical AI uses machine learning algorithms to analyze historical patterns across operational, behavioral, and experience data, generating predictive models that forecast future experiences and outcomes. A large university system could integrate student data streams – including course engagement metrics, support interactions, and direct feedback – to identify early warning indicators and predict individual retention risk, facilitating proactive, personalized interventions that simultaneously improve student success metrics and optimize resource allocation across support programs.
- Root Cause Analysis. Analytical AI employs causal analysis to examine interconnected patterns across multiple data sources, surfacing the underlying drivers of critical business and experience outcomes. A global manufacturer could analyze integrated data from satisfaction scores, product quality metrics, production systems, and employee training records to identify which combinations of processes, practices, and workplace conditions drive measurable improvements in both product quality and workforce engagement, enabling targeted investments in the highest-impact experience improvements.
- Journey Analytics. Analytical AI applies advanced pattern recognition and sequential analysis algorithms to map complex experience journeys, revealing both explicit pathways and hidden relationships across millions of interaction points. A technology company could analyze employee interactions throughout their lifecycle – from recruitment and onboarding touchpoints to collaboration platforms and HR systems – to identify success patterns, surface systemic friction points, and quantify the impact of different journey paths on engagement outcomes, allowing the HR team to design targeted improvements that optimize both employee experiences and retention.
Generative AI
Generative AI encompasses technologies that create new, contextually relevant content by learning patterns from existing information. These systems, primarily powered by Large Language Models (LLMs), can understand and generate human-like text, images, and other media based on patterns in their training data. Unlike traditional systems that retrieve or combine pre-written content, generative AI creates original outputs that dynamically adapt to specific contexts while maintaining consistency with brand and communication guidelines.
Applications for Generative AI in XM include:
- Virtual Assistants/Chatbots. Generative AI powers dynamic, contextual conversations that adapt in real-time to user needs and interaction history. An insurance provider could deploy an internal chatbot that helps claims agents get immediate answers to complex questions about policies, coverage, and procedures. The chatbot would understand context from claim details and past interactions to provide accurate guidance – helping agents confidently interpret coverage rules, understand policy exceptions, and follow correct procedures without needing to consult subject matter experts in other departments.
- Personalized Communications. Generative AI creates tailored communications that automatically adapt to each individual’s context and needs while maintaining the organization’s brand voice and quality standards. A retailer could improve customer engagement by generating personalized messages based on someone’s purchase history, browsing behavior, and stated preferences, calibrating product recommendations, promotional offers, and message timing to create more relevant interactions that improve customer satisfaction and conversion rate.
- Adaptive Experiences. Generative AI transforms rigid, standardized content into dynamic experiences that automatically adjust for different audiences and contexts, such as dynamic dashboards, digital interfaces, support flows, sales playbooks, and training materials. A financial services firm could accelerate new customer onboarding by generating tailored experiences that adapt based on each customer's declared preferences, financial goals, and expertise level, modifying things like product explanations, interface complexity, and educational content to create better experiences that improve both customer understanding and activation rates.
- Prescriptive Guidance. Generative AI translates complex data into clear, actionable guidance for specific roles and situations, like next-best-action recommendations, performance coaching, and operational alerts. A quick-service restaurant chain could improve employee experience by automatically generating targeted recommendations for store managers based on engagement metrics, operational data, and best practices. For example, “Your team's engagement scores are declining due to peak-hour understaffing. Schedule two additional staff members next week and hold a team meeting to address workload concerns." The system could then help draft related communications and meeting agendas to ensure effective follow-through.
Agentic AI
Agentic AI represents the next evolution in artificial intelligence – systems that combine advanced reasoning with orchestration capabilities to independently plan and execute complex tasks. Unlike other forms of AI that excel at specific functions like data analysis or content generation but require human intervention, Agentic AI systems can autonomously coordinate multiple processes to achieve broader objectives. These systems also go beyond traditional automation as, rather than rigidly following predefined workflows, they can understand context, develop flexible action plans, and dynamically adapt their approach based on real-time feedback, much like a human would. By managing routine processes and decisions independently, these systems enable employees to focus on more complex and meaningful work, like strategic planning, creative problem-solving, and delivering high-value interactions.
Applications for Agentic AI in XM include:
- Autonomous Issue Resolution. Agentic AI manages end-to-end problem resolution across multiple systems and touchpoints, adapting its approach based on context and feedback. An airline could deploy Agentic AI that transforms disruption management by continuously monitoring flight operations, weather patterns, and passenger experience signals. The system would independently manage recovery efforts – rerouting affected passengers, adjusting crew schedules, reallocating aircraft, and personalizing compensation offers – while learning from previous scenarios to optimize its resolution strategies based on factors like passenger status, connection impacts, and available alternatives.
- Process Orchestration. Agentic AI coordinates complex workflows across systems and departments, balancing multiple stakeholder needs and constraints. A pharmaceutical company could deploy Agentic AI that transforms new drug launches by coordinating across research, manufacturing, regulatory, and commercial teams. The system would independently manage launch activities – organizing clinical trial logistics, aligning production timelines, managing regulatory submissions, synchronizing training programs, and planning market access initiatives – all while adapting its decisions based on factors like trial results, regulatory requirements, and healthcare provider readiness to ensure successful, integrated product introductions.
- Proactive Support. Agentic AI monitors multiple data streams to identify early warning signals and initiates preventive interventions before issues impact experiences. An automotive manufacturer could deploy Agentic AI that transforms vehicle maintenance by analyzing patterns across sensor data, maintenance records, and driver feedback. The system would independently flag potential issues – identifying emerging mechanical problems, usage-based maintenance needs, or safety concerns – then coordinate preventive actions like scheduling service appointments, ordering parts proactively, or sending driver alerts, all while adapting its approach based on vehicle type, driving patterns, and owner preferences.
- Resource Optimization. Agentic AI directs critical resources to their highest-value uses based on performance data and business requirements. A consulting firm could deploy Agentic AI that transforms strategic staffing by continuously analyzing project requirements, consultant capabilities, and market opportunities. The system would independently optimize talent deployment – matching consultants to engagements, identifying skill development needs, and managing capacity planning – while adapting its strategies based on factors like client priorities, career development goals, and emerging market demands to maximize both consultant growth and business impact.
8 Steps for Incorporating AI into XM Efforts
While understanding AI categories and capabilities is valuable, insight without action doesn’t produce results. XM leaders need to be able to systematically evaluate, select, and implement the right AI solutions for their organization. And these use cases cannot be separate activities disconnected from their broader activities – they must be thoughtfully incorporated into your CX or EX program roadmap and aligned with existing priorities.
To help XM leaders move from understanding to implementation, we've developed a practical approach that connects AI capabilities to experience priorities. Think of the three AI categories we've explored as tools you can deploy to help evolve and enhance your Experience Management efforts. Our comprehensive report on "Driving Financial Impact through AI-enabled Customer Experience" shows how to systematically incorporate AI into core experience activities, from gathering insights to designing experiences to improving operations, using real company examples to bring recommendations to life. Taken together, the AI-enabled CX framework along with the three AI categories introduced here will help you understand both what AI can do and where to apply it for maximum impact.
Drawing from this research and conversations with organizations successfully deploying AI, we've identified eight steps XM teams can follow to move beyond pilots and capture meaningful value from these capabilities:
- Map potential AI use cases to XM priorities. Before evaluating specific AI solutions, start by clearly defining the experience and business outcomes you need to achieve. XM teams often make the mistake of selecting initial AI use cases based on technological novelty, executive pressure, or vendor promises rather than strategic alignment. But no matter how technically impressive your AI initiatives are, they won't scale or deliver meaningful value unless they align with your organization's strategic direction and experience priorities. Begin by identifying your most pressing XM challenges and priorities, whether tied to program objectives, high-friction journey points, operational bottlenecks, or roadmap projects. Next, identify key stakeholders, understanding both the roles of employees who will use these tools and how they interact with existing systems and processes. Finally, align specific AI capabilities with these opportunities, documenting how each could help you reach those goals more effectively or enable new capabilities that advance your XM maturity (e.g., using a CX or EX project evaluation inventory).
- Inventory available AI solutions. Before pursuing new AI investments, thoroughly assess the capabilities already available through your existing technology vendors and partners. XM teams often overlook valuable AI features already included in their current tools while pursuing new, standalone solutions that create additional complexity and integration challenges. Start by reviewing AI features available in your current XM platform and other key systems – many vendors have added sophisticated analytical, generative, and even agentic capabilities that you might not be fully utilizing. Document these capabilities alongside your priority use cases, noting where you might be able to activate new features through current contracts. Then identify any genuine capability gaps that might require new solutions. Use this comprehensive view of your current state to inform critical build versus buy decisions, considering factors like data privacy requirements, customization needs, and long-term scalability goals.
- Develop a structured evaluation framework. Before activating AI capabilities or pursuing new solutions, establish clear criteria for assessing and prioritizing different opportunities. XM leaders often struggle to compare diverse AI use cases objectively, leading to either analysis paralysis or decisions driven by the loudest voice in the room. A well-designed evaluation framework helps you systematically assess each opportunity's potential value and implementation complexity, ensuring informed decisions about where to start. Begin by defining key assessment criteria – like technical complexity, data readiness, organizational dependencies, potential value, regulatory requirements, and strategic importance. Then rate each of your potential AI use cases against these criteria, creating a clear picture of the risks and requirements associated with each opportunity. This might take the form of anything from a simple 2x2 matrix evaluating your top two criteria to a more robust CX or EX prioritization framework.
- Build a balanced portfolio of planned AI initiatives. Using your evaluation framework, identify a strategic mix of AI initiatives that balance quick wins with transformational opportunities. Organizations often make the mistake of either pursuing too many pilots simultaneously or betting everything on a single large-scale implementation. A balanced portfolio helps you build credibility while laying groundwork for transformation. Start by identifying quick wins – opportunities to activate readily available AI capabilities that can demonstrate immediate value with minimal risk, like using Analytical AI to automate survey analysis or Generative AI to summarize insights. Then select XM "lighthouse" projects – more substantial initiatives that can prove AI's potential, such as using a combination of Analytical and Generative AI to create a proactive customer retention program that identifies at-risk accounts and automatically generates personalized outreach. Finally, map out strategic initiatives that might require more foundational changes, like implementing Agentic AI to orchestrate end-to-end service processes across multiple departments and systems. While organizations can pursue multiple AI categories simultaneously, XM teams often start with Analytical AI to establish data practices and ROI models before moving to more complex Generative and Agentic AI applications. Remember that successful implementation requires early partnership with HR to understand and plan for how these initiatives will change roles and workflows.
- Create a business case for prioritized initiatives. Translating AI opportunities into funded initiatives requires demonstrating both financial and experiential impact in terms that matter to stakeholders across your organization. Too often, XM leaders focus narrowly on experience metrics when seeking support for AI investments, missing the opportunity to connect these improvements to broader business outcomes. A well-constructed business case helps you not only secure initial funding but also build momentum for future AI investments. Start by identifying relevant metrics across three categories: business impact (cost savings, revenue growth, risk mitigation), experience measures (satisfaction, effort scores, engagement levels), and operational indicators (adoption rates, accuracy levels, processing times). Document current baseline performance and project potential improvements. Be sure to highlight both direct experience benefits and broader business value, for example, showing how an initiative can reduce customer effort while simultaneously lowering support costs or how AI tools can help employees produce higher quality work more efficiently. For quick wins, focus on metrics that can demonstrate rapid ROI (e.g., reduced analysis time, improved response accuracy). For lighthouse projects and strategic transformations, develop more comprehensive frameworks that balance immediate gains with long-term value, defining clear milestones along the way (e.g., how an AI-powered retention program might reduce churn by 15% through early intervention while building capabilities for automated, personalized outreach at scale).
- Establish governance guidelines. Before implementing any AI initiatives, define clear policies and controls for how AI will be used within your XM program. Many organizations rush to implement AI capabilities without establishing proper oversight and employee enablement, creating risks around data privacy, ethical use, and quality control that can derail implementation and damage trust with both customers and employees. This is particularly critical in XM, where AI systems often process sensitive personal information and directly impact customer and employee experiences. Define policies across critical areas like data usage (what information AI can access and how it can be used), ethical guidelines (ensuring fair and transparent AI use), quality controls (accuracy thresholds and monitoring requirements), and human oversight (when and how humans review AI outputs). For quick wins, leverage existing governance frameworks where possible, like current data privacy policies or content review processes. For lighthouse and more strategic initiatives, work with legal, HR, IT, and risk teams to establish robust guidelines that address the unique challenges of sophisticated AI applications (e.g., agentic systems that make autonomous decisions), while setting clear expectations for employee usage and accountability.
- Roll out AI initiatives through focused pilots. Before scaling any AI initiatives, use well-designed pilots to validate approaches, manage risk, and justify broader implementation. Many organizations either make pilots too large and complex or fail to structure them in a way that generates meaningful learning. Start by selecting a specific use case with clear scope and success criteria. For quick wins, this might mean testing straightforward AI capabilities within existing processes, like automating survey analysis for one business unit, while lighthouse and broader strategic initiatives might involve testing more complex AI capabilities in a controlled environment with a targeted subset of users, like testing AI-enhanced employee onboarding within a specific department. Create a structured testing plan that defines what you're measuring, how you'll gather feedback, and what specific conditions would indicate success or signal needed adjustments. Your evaluation should examine technical performance (is the AI performing as expected?), human factors (are employees and customers comfortable with the solution?), and business results (are we achieving expected outcomes?). Document your learnings systematically to inform future initiatives and help make the case for scaling successful pilots across the organization.
- Create role-based employee enablement. Before fully launching an AI initiative, prepare the specific teams and individuals who will be using or affected by the new capabilities through structured training and support programs. Many organizations focus solely on technical deployment while underestimating the importance of user readiness and acceptance. Effective employee enablement ensures AI initiatives deliver value by helping people adapt to and embrace new ways of working. Using the stakeholder map you developed in the first step, create targeted engagement and training plans that connect each group's role to broader XM and AI objectives. Combine structured training with hands-on practice and ongoing reinforcement to build both capability and confidence. For quick wins, focus on training immediate users while establishing clear success behaviors. For lighthouse and strategic initiatives, develop more comprehensive enablement programs, which might include creating AI awareness campaigns, establishing leader advocacy programs, developing role-based learning paths, and launching peer champion networks.
The bottom line: Long-term XM success will require organizations to systematically incorporate three categories of AI into their customer and employee experience efforts.