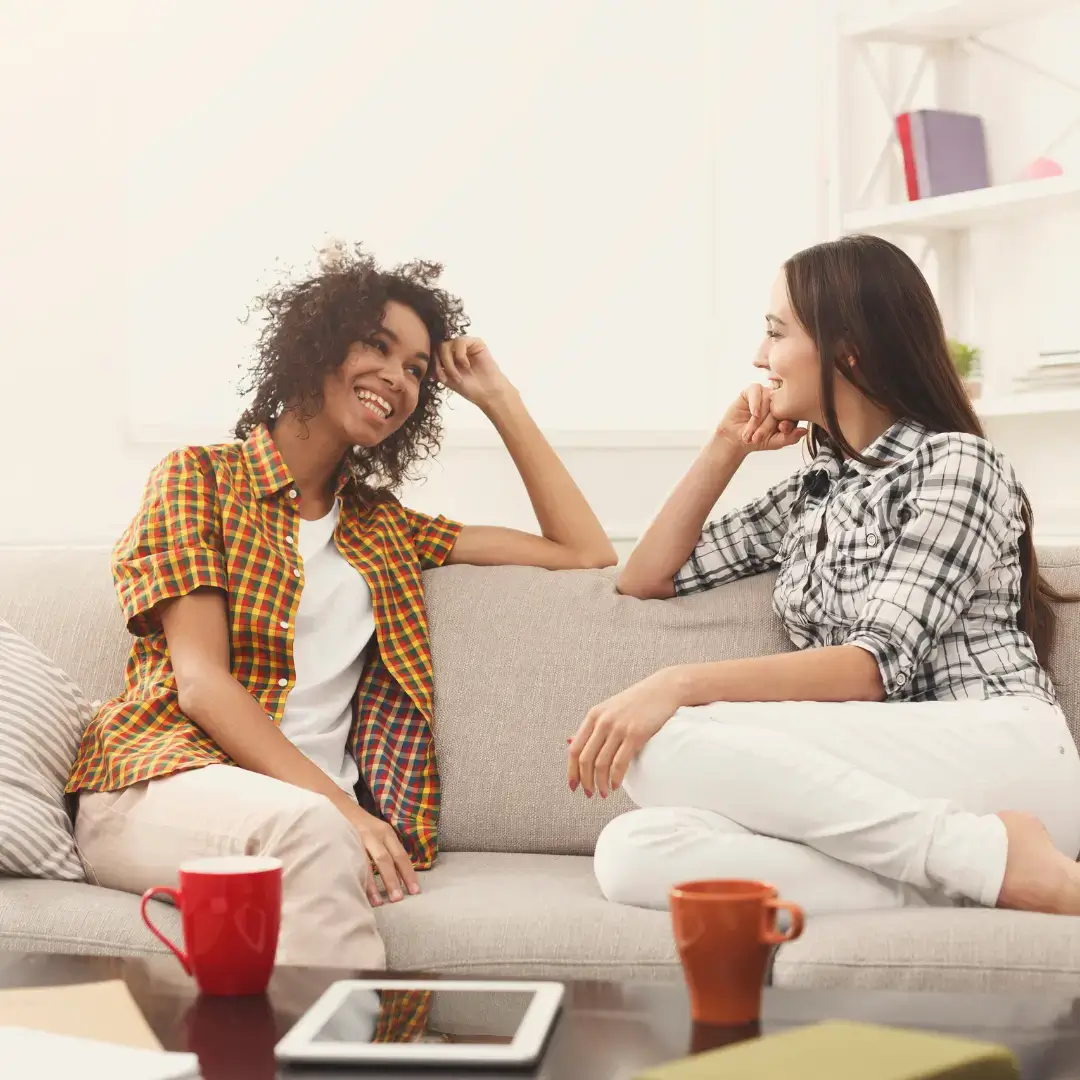
Customer Experience
Conversational AI: Exploring the future of customer experience management
Natural language processing, generative LLMs and virtual assistants… What do these industry buzzwords have in common? They all come under the umbrella of conversational AI – the process of turning human conversations into data, insights, and business-boosting impact. Here’s how…
What is conversational AI?
Conversational AI is the term given to artificial intelligence systems built to understand, analyze and participate in the nuances of human conversation. That’s tougher than it sounds; unpicking and applying meaning to the way we talk is an incredibly complex task, so it takes computer systems with deep large language models (LLMs) and natural language processing (NLP) capabilities.
You’ve probably seen more and more about conversational AI and LLMs in the news – and in the apps you use – over the past year or so, as generative AI tools and conversational AI chatbots like ChatGPT have opened up to the public.
Free eBook: Reimagining omnichannel CX in the age of AI
Conversational AI vs Generative AI: What’s the difference?
Generative AI is a subset of conversational AI. ‘Generative’ here means that the tool in question can generate written or spoken responses to conversational input. So you’ll see the term generative AI used to describe the latest generation of tools like ChatGPT, but these are still using conversational AI to understand what’s being asked of them.
The core difference is that you might have a conversational AI tool that doesn’t have generative AI capabilities – for example, an analytics suite that can understand customer conversations but isn’t designed to directly respond to them.
These conversational AI tools are driving a new era of computing, but they’re also enabling new ways of working in the customer experience space, where the ability to understand conversations at scale is supercharging how businesses design customer journeys, personalize experiences, and deliver customer support.
And that’s exactly what we’re going to cover in this guide…
AI Jargon Buster
Let’s quickly run through some of the terms in the conversational AI stable…
Generative Pre-trained Transformers (GPT)
These are a type of AI model that has been trained on large amounts of data and can generate human-like text, images, and even audio.
Large Language Models (LLM)
Similar to GPTs but are specifically designed for natural language tasks.
Natural Language Processing (NLP)
This is a subset of AI that focuses on analyzing and understanding human language.
Machine Learning (ML)
A technique used in AI where machines are trained to learn and improve on tasks without being explicitly programmed.
Neural networks
A type of machine learning model that’s inspired by the structure of the human brain that processes and transmits information, enabling models to learn and make predictions based on input data.
Deep learning
Deep learning is a subfield of machine learning that uses neural networks with multiple layers to solve complex problems such as recognizing patterns in data, making them well-suited for tasks such as image and speech recognition.
How does conversational AI work?
On the back end, conversational AI combines natural language processing and machine learning to create a complex model for human communication. That model starts by breaking down sentences into words, attributing meaning, and extracting key information.
That information is then cross-referenced against both a machine learning model – one that continually grows and learns as new information is added – and a static knowledge base of data, facts and rules about conversation generally works.
Think of it like learning to drive. You studied the driver’s manual so that when you’re out in your car, all those otherwise alien symbols on road signs can clue you in on what to expect and how to behave. And, as you drive more and more, your experiences on the road supplement that knowledge base to create a more capable, well-rounded driver who can handle anything the road has to offer.
On the front end, those smart capabilities are funneled into applications that provide value to the end user. That might be conversational AI chatbots and virtual assistants that can provide written answers to prompts, or experience management suites that can help customer support teams analyze and adapt to customer interactions.
What are the benefits of conversational AI?
Conversational AI has a bunch of business use cases, but they all fall under three key benefits: scale, speed, and interoperability.
Conversational AI: Scale
Conversational AI capabilities allow businesses to monitor and understand the context of customer communication at a previously unimaginable scale. Traditionally, this has meant manually analyzing data from small samples – and taking time away from other jobs to do so. AI can be on every call, read every social media post, and answer customer queries via instant messaging all at once, meaning you won’t miss an important signal among the noise.
Conversational AI: Speed
Automatic speech recognition is instant, which means it can do everything mentioned above in real time. For customer support teams, as an example, that allows for software tools that can deliver agents real-time prompts for how to resolve an issue, or real-time compliance scoring that escalates matters the moment things take a turn.
Conversational AI: Interoperability
The natural language understanding demonstrated by today’s best artificial intelligence tools is able to piece together information from across channels and touchpoints. For businesses, that means surfacing trends, patterns and insights about potential customer pain points from myriad sources. If you’re doing this manually, it’s impossible to connect the dots across channels without missing important markers and data points.
Crucially, all of these characteristics provide powerful methods for analyzing and improving the customer experience at every level, which is what will drive positive change to core metrics like customer satisfaction, churn, and retention.
Conversational AI challenges and controversies
What’s important to remember about anything AI-related is that the underlying technology isn’t magic – and it definitely doesn’t represent Skynet-level sentience. Instead, conversational AI is built on a network of pattern recognition, rules, and pre-existing content. And, while the resulting end uses of that kind of network can be very powerful, it does also have its limitations.
In generative AI chatbots in particular, these limitations can result in errors, misunderstandings, and ‘hallucinations’ – the term given to moments when a generative AI tool presents false information as fact or ‘forgets’ basic language rules. The other big issue facing large language models is that, because they are trained on existing material (often general information scraped from across the internet), there is always a risk that they’ll produce content that directly plagiarizes someone else’s work.
The answer in most of these cases is to apply a solid layer of common sense to whatever you’re using generative AI for – and to remember that these are tools for reaching a human-powered end, rather than flawless solutions in and of themselves.
For companies employing this technology, perhaps the biggest challenge is around privacy. After all, if you’re using AI to monitor conversations, you need to do so ethically. The solution here is to only use tools that have appropriate contractual agreements in place to secure both business and customer data, and that meet up-to-date industry and regulatory guidance.
Qualtrics has a great, free AI security and privacy guide that can help here.
Conversational AI use cases
In the form of generative AI chatbots, conversational AI technology can be put to work on a wide range of useful tasks, from crafting emails to supplementing search engine queries, and even as an extra perspective to bounce ideas off.
Businesses, on the other hand, can make use of conversational artificial intelligence tools to turn human language into insight that can help improve the overall customer experience – across a range of verticals.
Conversational AI and experience management go hand-in-hand because AI is great at scooping up information from every customer interaction at once and surfacing trends that might otherwise be missed.
Here are a few conversational AI capabilities that can help improve customer satisfaction:
Targeted coaching for agents
With natural language processing and real-time conversational AI tools, managers can get automatic alerts on areas where specific agents need help, alongside clips of conversations to review. Agents can also self-coach, since they get instant feedback on chats, can track their scores, and review parts of chats where they need to improve.
Real-time tracking of the customer experience
The right tools can help score each conversation and interaction against wider CX metrics, letting you see how those scores trend versus your ongoing goals. The data can be sliced by customer type, topic, and agent, helping you quickly spot trouble areas. If pain points in the customer experience start to arise, conversational AI will detect them by piecing together insights from your entire network of interactions – long before any human eye could possibly piece things together.
Keeping tabs on customer health
By combining conversational AI chat insights with other behavioral data, companies can grade their relationship with every customer. This can identify any issues that might be hurting customer health and find customers at risk of not renewing. This kind of proactive monitoring can also trigger outreach to critical customers in trouble.
Smart, continuous improvement
Natural language understanding, applied to chat topics, agent performance, and customer sentiment for every interaction, helps businesses prioritize areas for improvement. That might be where to invest in new systems, tools, and training, as well as how best to staff support teams.
Proactive customer recovery
If an AI platform spots a customer conversation that’s heading the wrong way (based on sentiment, effort, or agent behavior), it can trigger a range of automated responses, from automatic follow-up with the customer to real-time agent prompts that can help mitigate issues.
Active compliance monitoring
Conversational AI can also detect and act whenever sensitive agent behaviors or topics arise – instantly flagging issues, informing compliance monitoring teams, or triggering set workflows to help squash issues right as they arise.
Distributed signal detection
It’s important that stakeholders can access the insights that matter most to them – as they happen, and in a way that spans departments. That means that the best kind of conversational AI tool is one that can break down silos, make data transparent, and present the right actions to the right people. That could be product managers being made aware that customers are complaining about features, or pricing analysts being alerted to real-time chatter on competitor pricing.
Omnichannel intelligence
Customers routinely talk with and about brands across a raft of channels and touchpoints. Taking all these disparate data points, combining them, and turning them into insights is a near-impossible task for people, but it’s where conversational AI shines. With all these interactions brought into a single source of truth, you’ll be able to understand patterns and trends that show where gaps in the customer experience ought to be fixed, and what changes need to be made.
The Qualtrics difference…
More than 3.5 billion pieces of customer and employee feedback are collected and analyzed on the Qualtrics® XM (Experience Management) Platform® each year.
AI has always been a core part of that toolset, but with the recently deployed XM/os2, artificial intelligence is now more central than ever to our ability to transform brands’ customer experience.
XM/os2 can…
- Instantly generate summaries after every single interaction
- Automatically create support tickets
- Proactively recommend actions to agents
- Create summaries of employee experience data
- Find links between HR metrics and behavioral patterns
- Deliver personalized AI-driven coaching plans
- Build team-level insights and tailored recommendations
- Make insights available to everyone in the organization
- Identify fresh insights by bringing previously siloed datasets together
You can learn much more about XM/os2 here.
Free eBook: Reimagining omnichannel CX in the age of AI